Thought leaders are throwing around many AI trends in the business world. But don’t worry, this one is broad and easier to understand than others.
AI simulation combines AI techniques with virtual environments to train and test AI bots. AI adoption of this technology is crucial today. It speeds up innovation and problem-solving in all fields.
Private investment in global AI was $91.9 billion in 2022. AI simulation will drive much of the increase in AI investment in the coming years.
An example of AI simulation is within autonomous vehicle development. AI simulations replicate real driving scenarios. They let algorithms learn and improve without risking lives or expensive trials.
This process ensures that manufacturers can deploy safer and more reliable self-driving technology. This vision represents the AI-augmented workforce of the future as we all use these transport systems to get to work safely and efficiently.
Scroll down to have your mind enflamed by the possibilities of AI simulations. You will see the best use cases and types of AI simulation. These insights will let you improve how you use AI simulations. They will do so within your AI-driven digital transformation.
Use cases for AI simulation
Even if you understand AI simulation, it can still be hard to see how to use it in your organization. You must also adjust your tech adoption to get ROI for this new tech. It can potentially be one of the most influential AI productivity tools this year.
Let’s review some use cases. They will help direct you to use AI simulations, no matter what type of organization and technology adoption strategies you lead.
Fostering business acumen, encompassing data analysis and financial literacy
Simulations are immersive. They replicate real scenarios. These simulations also foster business acumen through AI. Participants do data analysis exercises. They learn to interpret and use data for strategic decisions.
Simulated tasks cover budgeting, forecasting, and financial modeling. They improve financial literacy. They let people fully understand financial concepts and their use in business.
The simulations are risk-free. They are for experimentation and learning. They help players refine skills and gain confidence. They do this by making sound business decisions based on data-driven insights.
Addressing business performance obstacles
Using AI simulations to fix business problems involves finding KPIs. Then, you study them. They show where to improve in a company.
Participants engage in scenario-based simulations. They use them to diagnose issues, develop strategies to overcome challenges, and implement solutions to improve performance.
The best approach is to train in advance and gradually experiment and learn from each goal passed. It is also helpful to offer the opportunity for user feedback. Feedback helps people. It helps them develop skills in analysis and strategy.
These are needed to address performance obstacles and drive positive change well.
Navigating complex leadership dilemmas adeptly
AI simulations facilitate adeptly navigating complex leadership dilemmas, which present participants with challenging leadership scenarios.
These simulations simulate various leadership challenges, such as managing conflicts, making tough decisions under pressure, and inspiring teams to achieve goals.
Participants are required to assess situations, consider multiple perspectives, and apply leadership principles to guide their actions effectively.
By engaging in these simulations, individuals develop critical thinking skills, emotional intelligence, and decision-making capabilities for navigating complex leadership situations confidently and competently.
Managing challenging conversations effectively
Managing tough conversations is a key skill. AI simulations can help leaders improve this skill. The simulations replicate real scenarios. Participants engage in tough conversations, giving feedback, resolving conflicts, and negotiating.
Participants get chances to practice active listening. They also practice empathy and communication skills. These skills help them navigate tough interactions with clarity and professionalism.
People enhance their ability to handle difficult conversations with confidence and achieve good outcomes. They do this through exposure to diverse conversation scenarios and feedback.
High-stakes sales and negotiation scenarios
Participating in high-stakes sales and negotiation scenarios through AI simulations offers a dynamic learning experience for individuals seeking to enhance their sales and negotiation skills.
Participants engage in simulated sales presentations, negotiations, and deal-making scenarios that mimic real-world business environments.
They develop sales strategies, communicate value propositions effectively, and negotiate favorable outcomes while navigating competitive pressures and stakeholder interests.
These simulations allow individuals to refine their negotiation tactics, build rapport with clients, and cultivate the resilience and adaptability required to succeed in high-pressure sales and negotiation situations.
Types of AI simulation
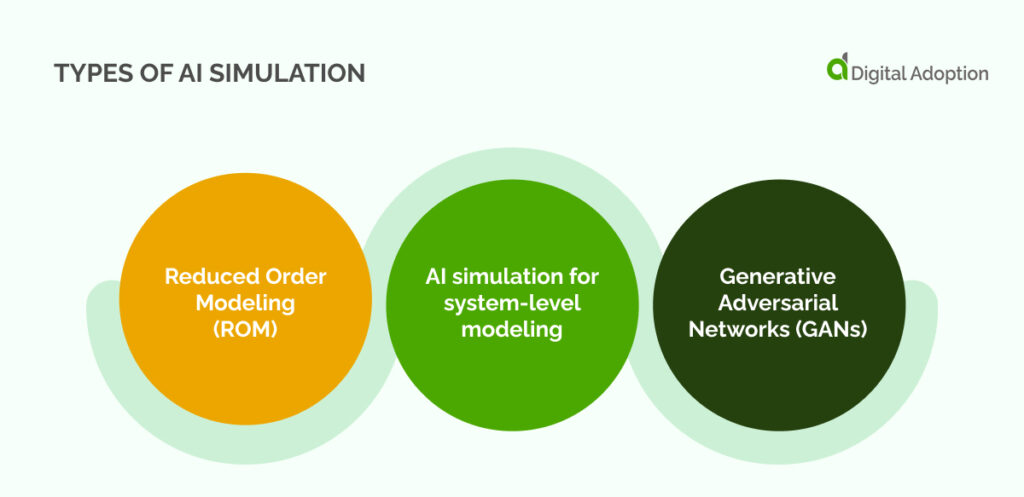
There are three main types of AI simulation. The simulations range from simple models to generative adversarial networks. They empower businesses to improve processes. They also help with product design innovation. And, they help with data-driven decisions. They ultimately drive success.
Understanding them is crucial for maximizing return on investment (ROI) and gaining a competitive edge in the enterprise landscape.
Reduced Order Modeling (ROM)
Reduced-order modeling (ROM) has long utilized physics-based mathematical matrices to simplify 3D system simulations, sacrificing some fidelity while remaining grounded in physics principles.
This tradeoff proves beneficial as the time saved outweighs the minor loss in accuracy. The innovation brought by AI, particularly neural networks and machine learning, lies in generating a machine learning model or “brain” from a limited set of simulation variations.
This model can then expedite subsequent simulations, surpassing the speed of traditional physics-based ROMs. Engineers can swiftly optimize designs using this approach. Acoustic, thermal, heat transfer, structural, and fatigue life simulations can all benefit from this data-driven methodology.
By harnessing AI, engineers gain a powerful tool for accelerating the iterative design process and achieving efficient solutions across various engineering domains.
AI simulation for system-level modeling
System-level modeling primarily focuses on 1D models to expedite simulations. When organizations apply AI in this context, leaders call it “system-level learning,” utilizing deep reinforcement learning techniques.
This method involves making a control system. It interacts with the 1D simulation model and runs many fast simulation sequences. There are often hundreds or millions.
An optimal control mechanism allows this self-play simulation. AI facilitates it. System-level modeling applications are commonly employed in control engineering and encompass various control systems, such as furnace temperature regulation.
Due to their minimal computational requirements, there’s a growing trend of utilizing these techniques on edge devices for industrial IoT applications.
This feature enables the resulting model to operate in real-time or near-real-time, making it particularly advantageous for time-sensitive industrial processes.
Generative Adversarial Networks (GANs)
GANs are key in early design. They help designers see their visions without building them in the real world with expensive materials.
GAN simulations generate many novel designs. These designs have a generator algorithm and discriminator algorithms. The discriminator subsequently scrutinizes them to distinguish realistic outputs from non-viable ones.
By effectively filtering out unrealistic designs, GANs facilitate the discovery of previously unexplored optimal design alternatives.
However, the efficacy of GAN simulations hinges on the availability of ample historical data from relevant domains. Formula 1 racing is a prime example of a GAN application, where racecar manufacturers amass copious amounts of data.
This data fuels virtual testing. It is used for computational fluid dynamics (CFD) to refine airflow patterns. In the conceptual design phase, historical data can be fed into GANs to fabricate realistic CFD models, guiding engineers toward innovative design solutions.
Why every business needs to invest in AI simulation
Data simulation proves invaluable for businesses of all sizes, offering multiple advantages in decision-making, risk assessment, performance evaluation, and model creation.
One primary benefit lies in aiding informed decision-making.
Organizations can explore multiple alternatives and analyze potential outcomes by simulating various scenarios mirroring real-world contexts. This step facilitates data-driven decision-making, minimizing uncertainty and increasing the likelihood of achieving desired results.
Another crucial point is that data simulation significantly enhances risk assessment and management. Organizations can simulate diverse risk scenarios, evaluate their probability and impact, and devise risk-mitigation strategies.
Businesses can identify vulnerabilities and analyze potential risk repercussions to implement effective risk management strategies to safeguard against threats.
Synthetic data is generated through simulation. It is crucial for developing and testing models. Organizations can train and test statistical or machine-learning models in controlled environments by creating synthetic datasets that closely resemble real data.
This step enables them to identify flaws, enhance model accuracy, and mitigate error risks before deploying the models in real-world scenarios.
Research the types of AI simulation before investing
Have you ever invested in a new technology without thoroughly researching it first?
If yes, you wouldn’t be the first organization to do so, and you probably won’t be the last.
Despite the excitement for this relatively new technology, only 43% of C-suites believe their leadership has enough AI skills to understand the risks and opportunities of AI.
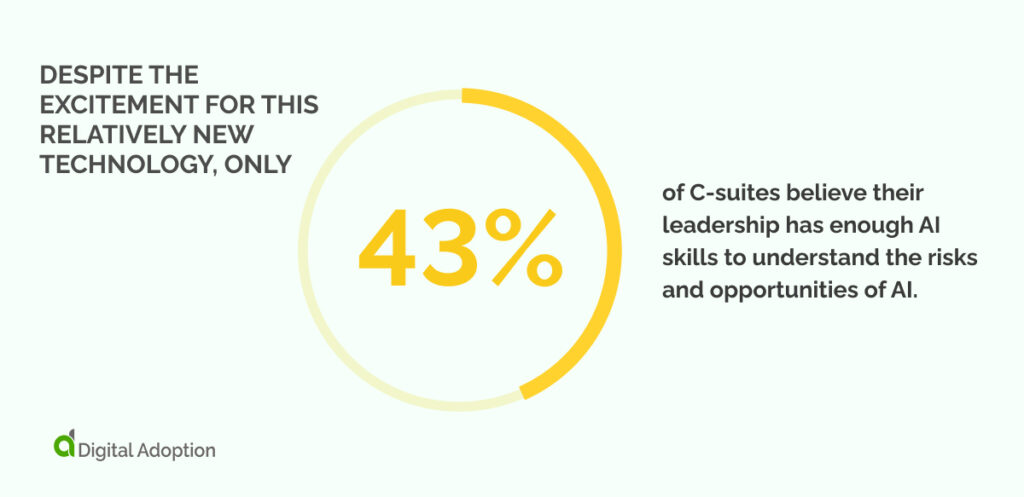
You must understand the types of AI simulation your organization needs. This is crucial before investing. It can reduce resource loss due to leaders not coordinating employee AI training.
It aligns simulation with needs. This action makes the investment more effective. Customizing AI simulation to fit industry requirements makes it more relevant and applicable. It makes integration smoother and maximizes ROI.
This knowledge empowers informed decision-making. It guides investments to the solutions. The solutions best address the organization’s challenges and goals.