AI is moving faster than ever, so you should know how different types work to get ahead of competitors within your digital transformation.
Conversational AI and generative AI are crucial elements in fulfilling various tasks and addressing customer requirements, yet they serve distinct functions and operate differently.
Conversational AI emulates human speech and allows humans to communicate with it using dialogue data sets. However, generative AI uses much larger data sets and can generate images, text, and sound.
The market value of AI rose by 46% to USD 207.9 billion. It is likely to grow each year thanks to the explosion in generative AI and the widespread adoption of conversational AI as organizations compete to optimize it in the most innovative ways to gain a larger share of their markets via digital adaptation efforts.
It’s essential to know these two types of AI, how they function, what use cases exist, and the ethical considerations of utilizing each to ensure your investment hits ROI.
What is the difference between conversational and generative AI?
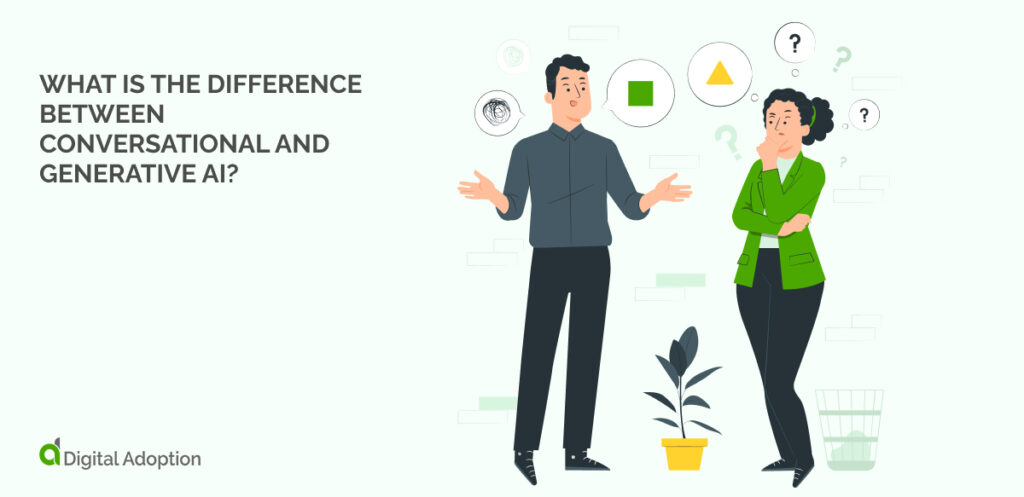
Let’s compare the most appealing AI trends, conversational and generational AI, by looking at each individually and then comparing the similarities and differences of the two.
Knowing these differences will help you choose the right technology for the right task and win in the marketplace as you optimize your investment to avoid waste and reduce employee frustration.
Let’s begin with conversational AI.
What is conversational AI?
Conversational AI technology facilitates more natural interactions between machines and humans, resembling conversations with two-way communication. It finds applications in chatbots, messaging platforms, and virtual assistants, with well-known examples including Alexa, Google Assistant, and Siri.
What is generative AI?
Generative AI empowers individuals to produce fresh content, spanning animation, text, images, and sounds, leveraging machine learning algorithms and the underlying training data. Utilizing deep learning and neural networks, generative AI generates outputs. Examples of generative AI applications include ChatGPT, Google Bard, and Jasper AI.
How does conversational AI function?
Conversational AI models undergo training on datasets containing human dialogues to grasp language patterns. Employing natural language processing and machine learning, they craft suitable responses to queries, effectively translating human conversations into machine-understandable languages.
The knowledge repositories conversational AI applications utilize benefit individual companies. Business AI software learns and expands its knowledge database through interactions, with humans also contributing updates to these repositories.
How does generative AI function?
Generative AI harnesses neural networks to discern patterns and structures within training data, generating fresh content based on predictions derived from these learned patterns. Various learning methodologies, including supervised learning, leverage human responses and feedback to enhance content accuracy.
Organizations can build foundational models as core AI systems for various tasks. Based on neural networks or machine learning, these models undergo rigorous training on extensive datasets. Their adaptability allows them to handle tasks like text translation, content generation, and image analysis. Examples include GPT-4 and PaLM 2.
How do conversational AI and generative differ?
Conversational AI and generative AI share many similarities but pursue distinct objectives, applications, training methods, and outputs. Each of these has unique capabilities shaping the future of AI, and how we use them will change over time.
Differences between these two AI technologies include:
1. Goals: Conversational AI prioritizes human interactions, while generative AI focuses on generating content across various formats.
2. Applications: Conversational AI finds utility in customer service, virtual assistance, and chatbots, while generative AI excels in crafting fiction, marketing materials, and meta descriptions.
3. Training data: Conversational AI learns from extensive datasets containing human interactions, queries, and responses, whereas generative AI trains on diverse datasets to discern patterns for content creation.
4. Input and output: Conversational AI responds to user input during conversations, leveraging interactions to formulate replies. In contrast, generative AI utilizes input and learned patterns to generate fresh content.
Primary Function | Creates fresh content across diverse formats such as poems, code, scripts, musical compositions, emails, letters, and more. | Mimics human dialogue by comprehending and engaging with user inquiries naturally and captivatingly. |
Focus | Originality and creativity. | Authenticity and human resemblance. |
Input | Written content, code, or alternative data. | Textual language or vocal communication. |
Output | Fresh, innovative textual content, imagery, and melodies. | Responses akin to human behavior in reaction to user inquiries. |
Training Data | Large datasets like the Internet. | Extensive collections of human dialogues. |
Applications | Content creation, images, research, coding, and many other forms of media. | Customer support, virtual aides, and conversational agents. |
Examples | ChatGPT, LaMDA. | Amazon Lex, IBM Watson Assistant, Google’s Dialogflow. |
Use case comparison
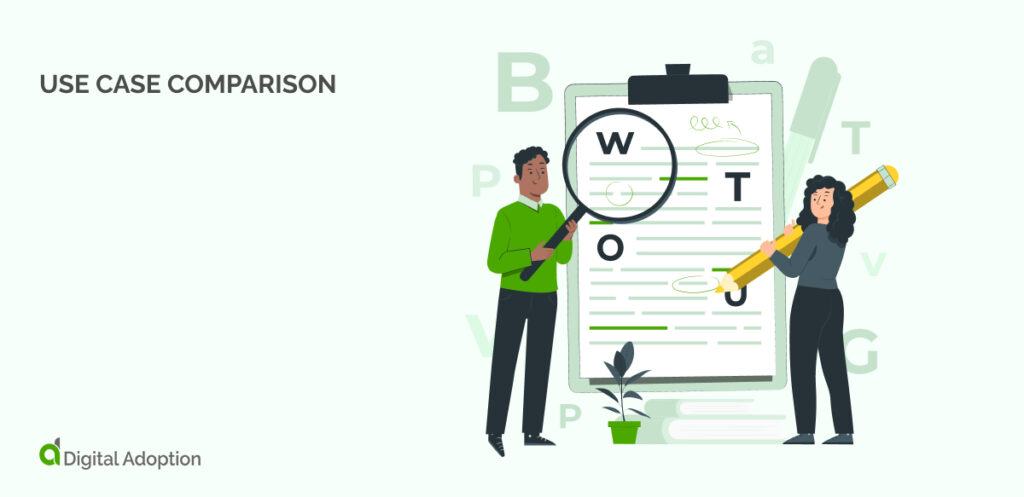
It can be challenging to separate conversational and generative AI into separate use cases. Generally, conversational AI is much more limited because it outputs dialogue only, while generative AI can generate text, images, and sound and uses training data in more detail. These features allow it to meet many more use cases than conversational AI.
Conversational AI use cases
Conversational AI can perform customer service, appointment scheduling, and FAQ assistance tasks in business. It is also helpful in retail, data collection, and IoT devices. Its ability to provide instant, personalized interaction dramatically enhances customer experience and efficiency.
Data collection
Conversational AI extends beyond customer engagement, offering data collection and analysis opportunities that inform strategic decision-making, giving your business a competitive advantage. Leveraging this data can enhance customer understanding and enable your staff to identify inefficiencies in existing processes.
Data collection use cases include:
- Capture all customer calls for documentation purposes.
- Enable search functionality to analyze interactions and identify areas for improvement in your contact or call center.
- Monitor calls using predefined keywords and phrases to understand common customer inquiries.
- Gather and assess diverse metrics such as call duration, daily call volumes, and call resolutions to enhance the customer experience in your contact center.
Retail
Conversational AI is suited to retail applications as customers increasingly use AI and expect AI to be part of their retail experiences.
Retail use cases include:
Product suggestions: Retailers can utilize conversational AI technology to generate product suggestions from customer interactions.
Cross-channel integration and scalability: Conversational AI platforms enable cross-channel communication, like web assistance, email, and messaging apps, improving conversions and sales and cutting costs compared to traditional methods.
Inventory management: Another practical application of conversational AI involves automatic inventory tracking to provide customers with real-time product availability updates.
IoT devices
Modern homes benefit from conversational AI devices, employing speech recognition for user interaction. These include Amazon Echo, Google Home, Google Assistant, Apple Siri, and Cortana. They enable users to interact with devices like they would talk to humans, quickly giving commands and initiating processes.
IoT device use cases include:
- Manage household devices using Google Home or Amazon Alexa.
- Monitor devices capable of calls or messaging.
- Amazon Alexa apps allow ordering food and learning preferences for personalized suggestions.
Conversational AI, though beneficial in many use cases, has its limitations. It still struggles with complex human language, context, and emotion and requires consistent updating and monitoring to ensure effective performance.
Generative AI use cases
Generative AI has many more use cases than conversational AI because it trains on broader data sets and can output various mediums, such as text, images, and sound.
These training methods allow generative AI to fit into many use cases, such as customer satisfaction improvement, workforce efficiency, content creation, and process optimization.
Optimize Customer Satisfaction
Generative AI for sales and other customer needs can improve satisfaction in several ways, including chatbots and virtual assistants to automate basic customer inquiries.
Generative AI can optimize customer satisfaction in the following ways:
- Chatbots and Virtual Assistants: Enhance customer self-service and lower operational expenses by automating responses to service inquiries with generative AI-driven chatbots, voice bots, and virtual assistants.
- Conversational analysis: Examine unstructured customer feedback from surveys, website comments, and call transcripts to pinpoint critical subjects, discern sentiment, and highlight emerging trends.
- Customization: Elevate personalized experiences and boost customer engagement through tailored offerings and communications.
Enhance workforce efficiency
Leveraging generative AI can revolutionize workforce efficiency, streamlining tasks and optimizing processes for enhanced productivity and organizational effectiveness.
Generative AI can enhance workforce efficiency via the following methods:
- Employee assistant: Enhance employee efficiency by swiftly accessing precise information, obtaining accurate responses, and generating or summarizing content via a conversational interface.
- Code generation: Facilitate application development by receiving code recommendations tailored to developers’ comments and existing code.
- Automated report generation: Leverage Generative AI to automatically create financial reports, summaries, and forecasts, streamline processes, and minimize errors.
Optimize creativity and content creation
Generative AI allows you to unlock creativity and streamline content creation processes through strategic optimization, harnessing the power of innovative tools and techniques that take a fraction of the time a human would be able to create them.
You can optimize creativity using the following methods:
- Marketing: Craft compelling marketing materials, including blog articles, social media posts, and email newsletters, to conserve time and resources.
- Sales: Produce tailored emails and messages using prospects’ profiles and behaviors, enhancing response rates. Develop sales scripts and discussion topics aligned with customer segments, industries, and offerings.
- Product Development: Utilize AI to create various design prototypes with specific inputs and constraints, expediting the ideation process. Alternatively, optimize current designs based on user feedback and defined parameters.
Support process optimization
Leveraging generative AI techniques enhances process optimization, offering innovative solutions for streamlining workflows and maximizing efficiency across various domains.
Support process optimization in the following ways:
Document handling: Enhance operational efficiency by automatically extracting and condensing data from documents and providing insights through generative AI-driven question answering. Explore further.
Data expansion: Create synthetic data for training ML models when the original dataset is limited, unbalanced, or sensitive.
Supply chain enhancement: Enhance logistics and cost-effectiveness by assessing and optimizing various supply chain scenarios.
Ethics for each AI type
Like any form of AI, it’s essential to consider the ethical implications of use for conversational and generative AI. When you know these ethical challenges, you can work to avoid them by adjusting how you use each form of AI to ensure you avoid these pitfalls.
Ethical considerations in conversational AI
Conversational AI systems must prioritize user data and conversation privacy and security during design and implementation. Additionally, businesses employing conversational AI chatbots must enforce strict controls to ensure compliance with relevant rules and regulations.
Ethical considerations for conversations AI include:
- Manipulative Marketing: Conversational AI can also deceive or manipulate users into purchasing products or services through misleading or coercive tactics.
- Spread of Misinformation: Unethical actors may deploy conversational AI to disseminate false information or propaganda, exploiting its ability to reach and influence large audiences.
- Privacy Violation: Conversational AI systems can unlawfully collect and exploit users’ data without their consent, leading to privacy violations and breaches of confidentiality.
- Social engineering attacks: Conversational AI can be employed in social engineering attacks to deceive individuals into disclosing sensitive information or performing actions that compromise their security.
Ethical considerations in generative AI (GAI)
GAI may occasionally generate biased or inappropriate content, necessitating designers to exercise heightened caution in training and continual improvement efforts to mitigate unethical responses.
Instances of generative AI risks and ethical ambiguities include:
- Misuse and manipulation: There are concerns about the potential misuse of generative AI for creating misleading or harmful content, such as deepfakes or manipulated images and videos, causing damage to organizational reputation.
- Bias and representation: Generative AI models can inadvertently perpetuate biases present in the training data, leading to biased or unfair outputs, particularly regarding representation and diversity in recruitment and hiring if it favors one ethnicity over another without the user being aware.
- Impact on employment: There are ethical considerations surrounding the potential impact of generative AI on employment, as it may automate tasks traditionally performed by humans, leading to job displacement and socioeconomic implications.
Consider the ethical considerations of generative and conversational AI to ensure you avoid pitfalls, and litigation and optimize your investment.
Generative AI is much more diverse than conversational AI
There is little overlap when you compare conversational and generative AI technologies in detail, as the features and use cases differ vastly.
For example, generative AI extends beyond conversational applications, encompassing tasks such as image synthesis, text generation, and creative content creation.
Unlike conversational AI, which focuses on dialogue, generative models generate new data, artwork, and even music, showcasing the breadth and versatility of AI’s creative capabilities across various domains and industries.
Ensure you choose the right technology for your AI-driven digital transformation to achieve the best results, meet your customers’ needs, and maintain financial sustainability.