Artificial intelligence (AI) is changing the world.
Generative AI has led to popular tools like ChatGPT and Google Gemini. Agentic AI takes things further, offering advanced solutions for big businesses.
Even with AI’s potential, there are still questions about how it works, especially how it interprets data to give results.
Large language models (LLMs) support many AI solutions today. They use natural language processing (NLP), natural language understanding (NLU), and deep learning to learn and understand data.
It is important to know how AI arrives at its final answers. To help with this, data analytics officers use Chain of Thought (CoT) prompting, which shows the steps behind LLMs’ answers.
This article explains Chain of Thought prompting—what it is, how it works, key techniques, and examples from different fields.
What is Chain of Thought prompting?
Chain of Thought (CoT) prompting is an AI method that clarifies a model’s decision-making process.
It helps AI systems break down complex tasks into simple steps, making their reasoning easier to follow. By doing this, CoT prompting helps AI give more accurate and reliable results since each step can be reviewed and improved.
This method is especially useful for AI models using large language models (LLMs), where knowing how the AI makes decisions is crucial for trust and effectiveness.
The problem
Traditional AI prompting often leads to results that are not transparent and lack consistency.
It’s not always clear how large language models (LLMs) reach their conclusions, which can lead to unreliable or wrong results.
These models usually generate text based on patterns in data without fully understanding the context or reasoning.
This can be a problem, especially for complex, multi-step tasks. Without a logical path, the AI’s responses might seem correct but lack depth, accuracy, or relevance.
The solution
Chain of Thought (CoT) prompting solves this problem by guiding AI through a step-by-step reasoning process.
Instead of producing answers in one step, CoT prompting encourages the AI to break down complex tasks into smaller, manageable parts. This allows the model to process information in order, making its reasoning clear and logical.
By clearly showing each step in the thought process, CoT prompting ensures that the AI’s output is correct and based on solid logic.
This approach greatly improves the reliability and accuracy of AI responses, making it better for complex tasks and decision-making.
How does Chain of Thought prompting work?
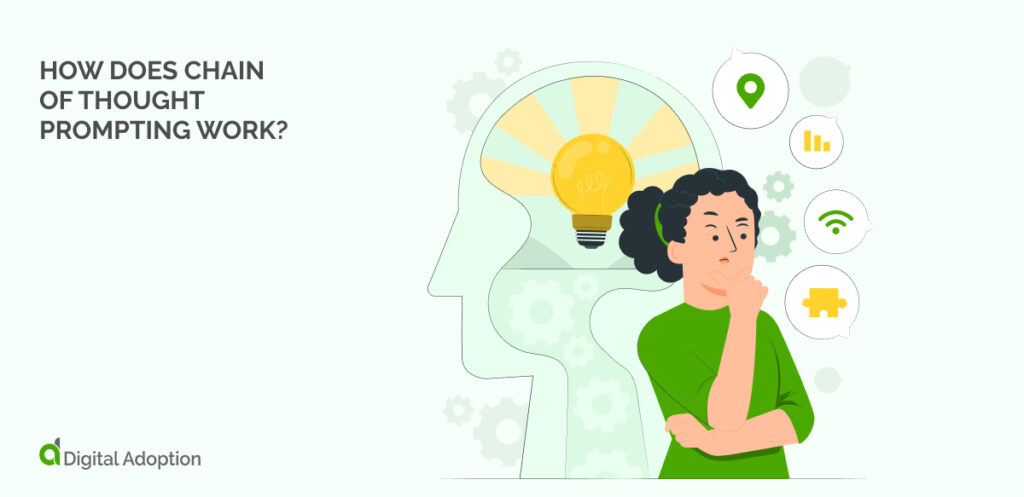
Chain of Thought (CoT) prompting improves the reasoning of AI models, especially large language models (LLMs).
It guides the model to break down complex tasks into simple, step-by-step processes. Instead of giving a single, unclear response, the AI is prompted to explain its reasoning, creating a “chain” of thoughts that lead to the final answer.
CoT prompting involves instructing the AI to think about each part of a problem one at a time. This helps the AI keep context and build a logical, clear response.
For example, when facing a multi-step problem, the model is encouraged to outline the problem, identify relevant information, and combine this information into a solution.
CoT prompting explains each step in the AI’s thought process, improving accuracy and transparency. This makes it easier to understand and trust how the AI reached a particular conclusion.
Chain of Thought prompting techniques
Here are some effective Chain of Thought (CoT) prompting techniques:
Automatic Chain of Thought (Auto-CoT)
Automatic Chain of Thought (Auto-CoT) automates the creation of reasoning steps in AI models.
Instead of needing manual input to create these steps, Auto-CoT lets the AI automatically generate a sequence of thoughts that lead to the final output. This makes it more efficient, especially for large-scale tasks where manual CoT prompting is not practical.
Auto-CoT is useful for complex problems or detailed analyses, as it allows the AI to generate logical reasoning paths on its own.
Zero-Shot Chain of Thought (Zero-Shot CoT)
Zero-Shot Chain of Thought (Zero-Shot CoT) prompts the AI to create a reasoning process without prior examples or specific training.
This method challenges the AI to think critically and produce a logical sequence of steps on its first try. Zero-Shot CoT is helpful when there is little training data or when the model needs to generalize across different tasks.
By encouraging the AI to reason independently, Zero-Shot CoT helps it handle new or unfamiliar challenges more effectively.
Few-Shot prompting vs. Chain of Thought (CoT)
Few-Shot prompting gives the AI a few examples of a task to guide its output.
This helps the model learn from limited data to produce accurate results. In contrast, Chain of Thought (CoT) prompting focuses on breaking down problems into logical steps, no matter how many examples are provided.
Few-Shot prompting improves the model’s ability to learn from periodic examples, while CoT enhances the model’s reasoning skills.
CoT is particularly useful for complex tasks that need detailed thinking, whereas Few-Shot prompting works better for tasks where context comes from examples.
Standard prompting vs. Chain of Thought (CoT)
Standard prompting directs the AI to give an answer based on simple instructions, often without explaining its reasoning. This method works for simple tasks but can lead to shallow or incorrect results for more complex ones.
Chain of Thought (CoT) prompting guides the AI in explaining a logical sequence of steps that lead to the final answer. CoT makes AI outputs clearer and more reliable by ensuring each step is well-reasoned and connected.
Standard prompting is good for basic tasks, but CoT is better for tasks needing deep reasoning and analysis.
Examples of Chain of Thought prompts
Here are some examples of Chain of Thought prompts in key business areas:
Example 1: Market entry strategy
Prompt: “An electric vehicle startup plans to enter a new market. List the key factors to consider when developing a market entry strategy. Include market analysis, competitive landscape, regulatory requirements, and potential barriers to entry. Explain the reasoning behind each factor, focusing on how these elements contribute to a successful market entry.”
Chain of Thought:
- Start by identifying target market demographics and buying behaviors.
- Analyze competitors, focusing on their market share, pricing strategies, and customer base.
- Assess regulatory requirements like safety standards and environmental rules that could affect market entry.
- Consider barriers like supply chain issues or cultural differences affecting product acceptance.
Example 2: Customer churn reduction
Prompt: “A SaaS company is experiencing a high customer churn rate. Identify the possible reasons for this churn and suggest strategies to reduce it. Include factors such as product usability, customer support quality, pricing structure, and competitor activity. Provide a logical sequence of steps to diagnose and address the issue.”
Chain of Thought:
- Start by analyzing customer feedback to find common problems, such as product usability or poor customer support.
- Examine the pricing structure and compare it with competitors to see if cost drives customers away.
- Look into recent competitor activities that might be attracting your customers.
- Recommend improvements, like better user experience, pricing adjustments, or improved customer support.
Example 3: Supply chain optimization
Prompt: “A manufacturing company is facing challenges in its supply chain. Propose strategies to improve supply chain resilience. Include considerations such as supplier diversification, inventory management, logistics efficiency, and risk mitigation. Break down the reasoning behind each proposed strategy and its expected impact on the overall supply chain.”
Chain of Thought:
- Look at the current reliance on single suppliers and suggest diversifying suppliers to reduce risk.
- Review inventory management practices to find inefficiencies and recommend just-in-time inventory techniques.
- Assess logistics processes to find areas for cost reduction and speed improvement.
- Implement risk management strategies, like planning for supply chain disruptions or finding backup suppliers.
Example 4: Business process improvement
Prompt: “A company wants to enhance its customer service efficiency. Propose a series of process improvements that could achieve this goal. Consider factors like response time, employee training, automation, and customer feedback systems. Explain the logic behind each improvement and how it contributes to better customer service.”
Chain of Thought:
- Start by reviewing current response times and identifying delays in customer service.
- Recommend better training programs for customer service reps to improve their problem-solving skills.
- Introduce automation tools like AI chatbots to handle routine questions, freeing up human agents for complex issues.
- Set up a customer feedback system to monitor service quality and find areas for further improvement.
The potential of Chain of Thought prompting
Building trust and using AI ethics responsibly is crucial for explainable AI.
Chain of Thought (CoT) prompting is important because it clarifies the AI’s reasoning process. Understanding how an AI model reaches its conclusions helps avoid significant risks.
Opaque AI decisions can lead to unintended issues, such as biased results, incorrect predictions, or hard-to-justify decisions. These risks are especially serious in healthcare, finance, and legal decisions, where mistakes can be costly.
With CoT, AI can solve more complex problems accurately, offering clear and logical insights.
As AI evolves, CoT prompting will likely become a key part of developing powerful and accountable AI models.
FAQs
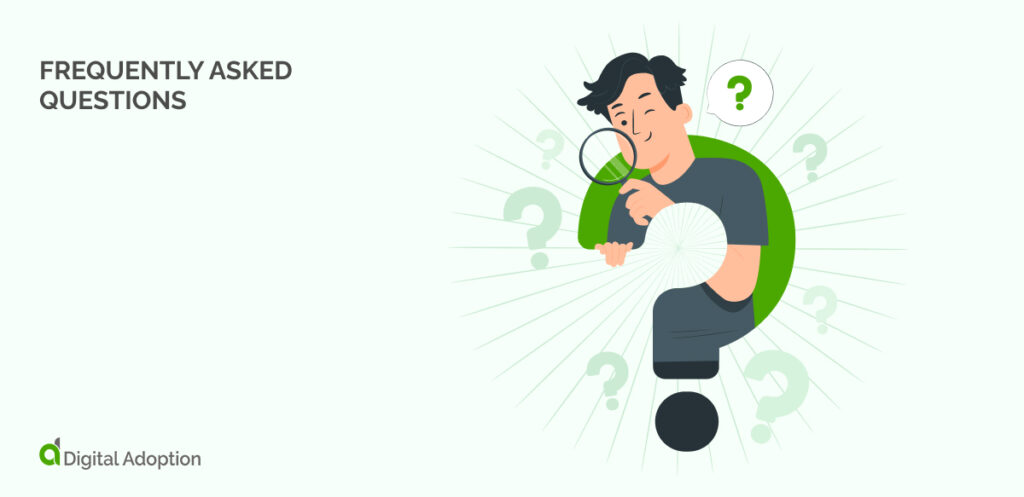
What industries can benefit the most from Chain of Thought prompting?
Chain of Thought prompting can help these industries by improving AI’s problem-solving and decision-making:
- Healthcare: Better diagnostics and treatment plans.
- Finance: Improved risk analysis and investment strategies.
- Legal: Enhanced case analysis and decision-making.
- Education: More personalized learning and tutoring.
- Research & Development: Efficient hypothesis generation and testing.
How does Chain of Thought prompting differ from traditional AI prompting methods?
Chain of Thought (CoT) prompting breaks down complex tasks into logical steps, while traditional AI prompting may not provide a clear reasoning process. CoT helps ensure each step in the reasoning is understood and validated, making AI responses more accurate and reliable.
Can Chain of Thought prompting be used with all AI models?
CoT prompting is most effective with large language models (LLMs) that can process and generate natural language. It can be adapted to other types of AI models, but its benefits are most visible in models that handle complex, multi-step tasks.