Since one of the biggest AI trends in decades, ChatGPT-4, was released in March 2023, there has been a rush to implement AI into every aspect of business, and now this is happening with business models.
We are now seeing an explosion of interest in cloud-based companies (IaaS, PaaS, and SaaS) providing various AI-based services. The need for these services is growing as enterprises rush to secure their AI-augmented workforce.
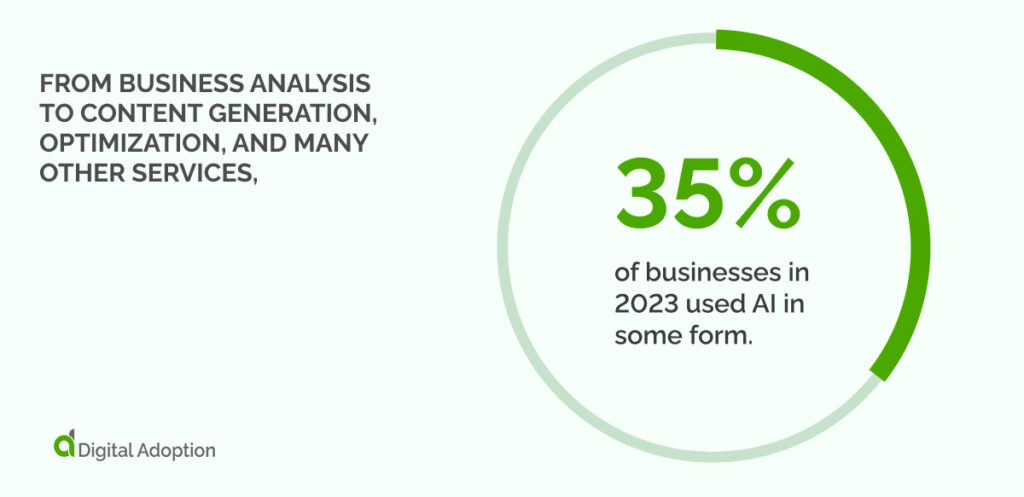
Therefore, now is the best time to consider AI business models to compete effectively in an increasingly challenging marketplace.
This article will delve into how AI business models are reshaping enterprises. The exploration will cover the definition of AI business models, their transformative impact on enterprises, the integration of AI into various business model types, the characteristics of a successful AI business model, and real-world use cases demonstrating the practical application of AI in diverse business scenarios.
What are AI business models?
AI business models are strategies that enterprises use to leverage artificial intelligence to increase efficiency and revenue.
These models encompass various approaches, such as subscription-based services, pay-per-use models, and selling AI-powered products. Companies may also monetize data generated by AI applications.
How are AI business models revolutionizing enterprises?
AI business models are revolutionizing enterprises as more organizations accept that they must implement AI using an overarching business model that impacts every process, operation, and employee task to ensure efficiency and fast ROI. The first aspect of this revolution is knowledge and data.
Knowledge and data
Data is the foundation of AI models. It includes structured and unstructured information that, when processed, forms the knowledge base. In AI business models, big data adoption enables informed decision-making, customer personalization, and operational efficiency.
Big data
Large volumes, often called big data, are crucial for training robust AI models.
In business, massive data allows for comprehensive analysis, pattern recognition, and predictive modeling, enhancing the accuracy and effectiveness of AI applications.
Data knowledge and understanding
Understanding data involves extracting meaningful insights. In AI business models, comprehending and interpreting data is vital for making informed decisions, predicting trends, and optimizing strategies based on a deep understanding of the business environment.
Graphs learning
Graph learning involves understanding relationships within data, which is valuable in various business contexts. In AI business models, graph learning can enhance recommendations, fraud detection, and network analysis, providing a more nuanced understanding of complex relationships.
Synthetic data
Synthetic data, artificially generated but mimicking real-world patterns, is helpful in scenarios where access to actual data is limited or sensitive. In AI business models, synthetic data aids in training models, ensuring robust performance without compromising privacy or security.
Knowledge representation
Knowledge representation involves structuring information to facilitate effective problem-solving and decision-making. In AI business models, proper knowledge representation enhances the system’s ability to understand, reason, and apply knowledge, contributing to more intelligent and context-aware operations.
Safe human-AI interaction
Ensuring safe interaction involves designing AI systems that consider human well-being. In business models, this includes creating user-friendly, transparent, human-centered AI interfaces that align with ethical guidelines to promote trust and adoption.
Agent symbiosis
Agent symbiosis focuses on collaborative interactions between AI systems and humans. In business models, this collaboration enhances productivity, decision-making, and innovation, creating a synergistic relationship between AI and human agents.
Ethics and fairness
Ethical considerations are crucial in AI business models to ensure fair and unbiased outcomes. Addressing bias, discrimination, and fairness issues is essential for building trust and maintaining a positive societal impact.
Explainability
Explainability in AI models is crucial for transparency. In business, understanding how AI reaches decisions fosters trust, aids compliance with regulations, and allows users to comprehend and trust the system’s outputs.
Trusted AI
Building trust ensures AI systems’ reliability, security, and ethical behavior. In business models, establishing trust is essential for user acceptance, stakeholder confidence, and the successful implementation of AI technologies.
Secure and private AI
Along with the power of AI comes severe security and privacy concerns. Organizations seek to improve their data security in the ways found below to ensure they reap AI’s benefits without becoming vulnerable to attacks.
Privacy
Privacy is crucial in AI business models to protect sensitive user information. Businesses must adhere to privacy regulations and build trust with users by ensuring their data is handled securely and ethically in AI applications.
Cryptography
Cryptography is vital in securing data and communications in AI business models. It encrypts sensitive information, ensures confidentiality, prevents unauthorized access, and protects user and proprietary business information.
Secure Multi-Party Computation
Secure multi-party computation allows parties to jointly compute a function over their inputs while keeping them private. In AI business models, this technique facilitates collaboration on data analysis without sharing raw data, ensuring privacy in multi-stakeholder environments.
Federated Learning
Federated learning decentralizes the training of AI models, allowing them to learn from data distributed across devices without centralizing sensitive information. This approach is crucial in AI business models where privacy concerns may arise, such as in healthcare or financial sectors, enabling model training without exposing raw data.
How AI works in business model types
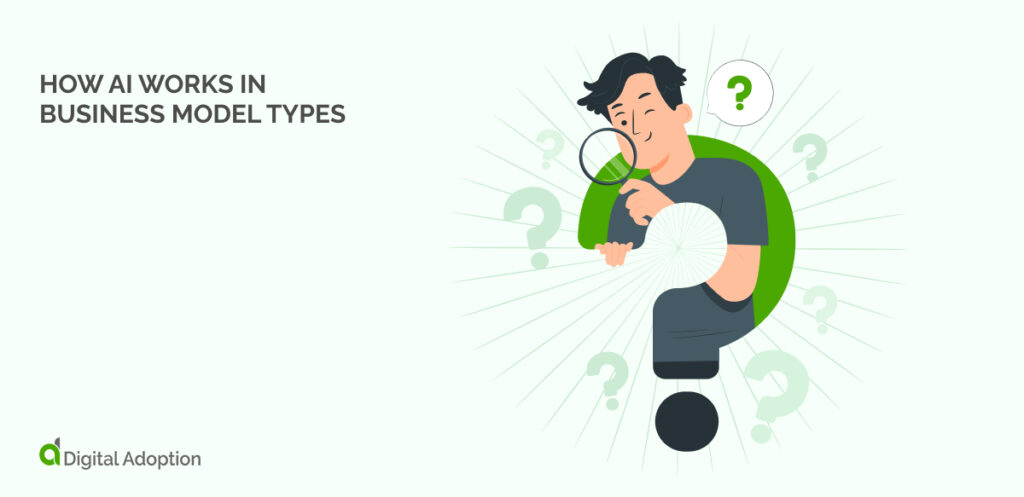
AI transforms business models from personalized customer experiences to streamlined operations. Explore its role in diverse models, shaping innovation and efficiency below.
Retailer
AI can enhance the retailer model by optimizing inventory management, predicting customer preferences, and personalizing the shopping experience. Machine learning algorithms can analyze historical data to forecast demand, reducing excess stock and preventing shortages.
AI-driven recommendation engines using ChatGPT sales techniques can provide personalized product suggestions to customers, leading to higher sales figures as part of a customer experience transformation.
Manufacturer
AI can be integrated into manufacturing to automate production processes, increasing efficiency and reducing costs. Predictive maintenance powered by AI algorithms can prevent equipment breakdowns, minimizing downtime.
Machine learning can optimize supply chain management by predicting raw material needs and ensuring timely procurement. AI-driven quality control systems can identify defects quickly, improving overall product quality.
Fee-for-service
AI applications in fee-for-service models can include automating routine tasks and freeing human resources for more complex and specialized activities. Machine learning algorithms can analyze data to provide valuable insights, enhancing service quality.
AI-powered virtual assistants can handle customer inquiries and support, ensuring efficient communication and service delivery.
Subscription
AI can play a crucial role in subscription-based models by analyzing customer behavior to predict churn and implementing personalized retention strategies.
Recommendation systems powered by machine learning can suggest additional services or upgrades based on individual preferences. Predictive analytics can optimize pricing models and identify customer segments’ most attractive subscription packages.
Freemium
AI can enhance freemium models by analyzing user behavior to understand preferences and tailor upgrade offers. Personalized content recommendations, powered by machine learning algorithms, can entice freemium users to explore premium features.
Chatbots equipped with natural language processing capabilities can engage users, providing valuable information about premium offerings and addressing user queries.
Bundling
AI can optimize bundling strategies by analyzing customer purchase patterns and preferences. Recommendation systems can suggest complementary products based on individual buying history, increasing the likelihood of successful bundling.
Machine learning algorithms can dynamically adjust pricing incentives to maximize the attractiveness of bundled offerings.
Marketplace
AI can improve marketplaces by implementing advanced search algorithms, enhancing user experience, and facilitating quicker transactions.
Machine learning can analyze user behavior to provide personalized product recommendations, increasing engagement and conversion rates. AI-driven fraud detection systems can enhance security and trust within the marketplace.
Affiliate
AI can optimize affiliate models by analyzing data to identify high-performing marketing channels and strategies. Machine learning algorithms can assess the impact of influencers and recommend partnerships with those with the highest conversion rates.
Automated analytics tools can provide real-time performance insights, helping companies make data-driven decisions in their affiliate marketing efforts.
Razor blade
In the razor blade model, AI can optimize inventory management, predicting when customers will likely need replacements.
AI-driven marketing campaigns can target customers with personalized promotions for disposable components, maximizing sales. Chatbots can offer product information and assistance, enhancing the overall customer experience.
Reverse razor blade
AI can support reverse razor blade models by analyzing customer preferences and behavior to tailor upfront product offerings. Predictive analytics can identify the most effective companion products to provide alongside the high-margin item.
Machine learning algorithms can optimize pricing strategies to maximize the upfront sale while ensuring customer satisfaction with the provided companions.
Franchise
AI can assist franchise models by providing data-driven insights for site selection and helping identify locations with high potential.
Machine learning algorithms can analyze market trends and customer demographics to optimize marketing strategies for each franchise location. AI-powered chatbots can enhance customer support for franchisees, providing timely assistance and information.
Pay-as-you-go
Organizations implement AI in pay-as-you-go models by tracking usage patterns and adjusting pricing dynamically. Predictive analytics can forecast peak usage times and enable companies to optimize resource allocation.
Machine learning algorithms can identify usage trends, helping companies refine their service offerings and pricing structures.
Brokerage
AI can streamline the matchmaking process between buyers and sellers in brokerage models by analyzing preferences and historical data. Natural language processing can enhance communication between parties, facilitating smoother deal negotiations.
Machine learning algorithms can assess market trends, providing brokers valuable insights for making informed decisions.
Consider these different types of business models and use them to adapt your existing model to accommodate AI and augment it effectively.
What makes a good AI business model?
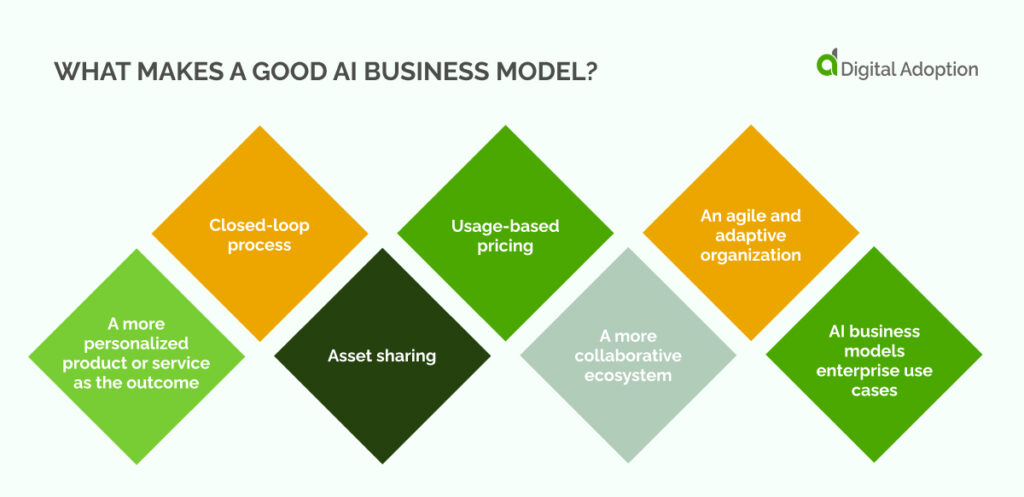
Before you build a new business model or adapt an existing one to accommodate AI, consider the points below for what makes the best AI business model for any organization.
A more personalized product or service as the outcome
An effective AI business model can provide customers with a more personalized product or service in the ways listed below.
Enhanced customer experience
Personalization tailors products or services to individual preferences, enhancing the customer experience. AI algorithms analyze customer data, behaviors, and preferences, allowing businesses to deliver tailored recommendations and content, increasing customer satisfaction and loyalty.
Increased Engagement
Personalization fosters engagement by providing relevant and targeted interactions. Doing so can lead to higher conversion rates as customers are more likely to respond positively to offerings that align with their needs and preferences.
Competitive Advantage
Businesses that offer a more personalized experience often gain a competitive edge. AI-driven personalization allows companies to stand out in a crowded market, attracting and retaining customers seeking tailored solutions.
Closed-loop process
The best AI business models include a closed-loop process to ensure users follow every step in a method and repeat as necessary. See below for more details on this process.
Continuous Improvement
The closed-loop process involves collecting feedback, analyzing data, and making iterative improvements. AI systems can automate this feedback loop, allowing businesses to refine their processes based on real-time data and insights constantly.
Efficiency and Effectiveness
Closed-loop processes minimize delays and streamline decision-making. By closing the loop between data collection and implementation of insights, businesses can quickly adapt to changing circumstances and optimize operations for efficiency and effectiveness.
Adaptability
The closed-loop approach ensures that businesses remain adaptable, responding promptly to market changes and customer feedback. This adaptability is crucial for staying competitive and meeting evolving customer expectations.
Asset sharing
Asset sharing is integral to the AI business model process. It encourages collaboration and sharing learning to ensure every organization member benefits from new knowledge in the ways written below.
Resource optimization
Asset sharing, facilitated by AI-driven platforms, allows businesses to optimize resource utilization. This action could involve sharing physical assets like office spaces or digital assets like computing resources. AI algorithms can efficiently manage and allocate shared resources based on demand, reducing waste and costs.
Collaborative opportunities
Asset sharing promotes business collaboration, fostering community and collective success. AI can facilitate the seamless sharing and coordination of assets, unlocking collaborative opportunities that benefit all involved parties.
Sustainability
Shared assets contribute to sustainability by reducing the need for redundant resources. AI can monitor and manage shared assets to ensure optimal usage and minimize environmental impact.
Usage-based pricing
Pay-per-use pricing in AI models offers cost flexibility, aligning expenses with actual usage, promoting scalability, and optimizing resource efficiency.
Flexibility for customers
Usage-based pricing models offer customers flexibility, allowing them to pay for the specific resources or services they consume. AI systems can track usage patterns, enabling accurate billing and preventing overcharges or undercharges.
Cost efficiency
Businesses benefit from usage-based pricing by aligning revenue with actual consumption. AI analytics can optimize pricing strategies based on usage data, ensuring fair pricing for customers while maximizing profitability for the business.
Scalability
Usage-based pricing scales with the customer’s needs. AI-driven systems can automatically adjust pricing tiers, making it easier for businesses to accommodate varying demand levels and allowing customers to scale their usage without friction.
A more collaborative ecosystem
A collaborative ecosystem in AI models fosters innovation, expands reach through partnerships, and enhances resilience through collective problem-solving and resource-sharing.
Acceleration
A collaborative ecosystem encourages knowledge-sharing and innovation. AI facilitates collaboration by providing platforms for shared data, insights, and resources. This process accelerates innovation as diverse stakeholders contribute to problem-solving and idea generation.
Expanded reach
Collaborative ecosystems extend businesses’ reach by leveraging multiple partners’ strengths. AI can connect different entities seamlessly, enabling efficient communication, resource sharing, and joint initiatives that benefit the entire ecosystem.
Resilience
businesses can collectively navigate challenges and disruptions in a collaborative ecosystem. AI tools can enhance coordination, communication, and adaptability, making the ecosystem more resilient to changes in the market or external factors.
An agile and adaptive organization
An agile organization in AI adapts swiftly to market changes, employs efficient decision-making, and cultivates continuous learning for sustained competitiveness.
Rapid Response to Change
Agility is the ability to respond quickly to changes in the business environment. AI-driven analytics and decision-making systems empower organizations to swiftly adapt strategies, operations, and products in response to market shifts, technological advancements, or customer preferences.
Continuous learning
AI supports an organization’s continuous learning culture. Machine learning algorithms analyze data and provide insights, helping teams understand performance metrics, customer behaviors, and market trends. This knowledge informs ongoing improvements and adaptations.
Efficient decision-making
An agile organization relies on efficient decision-making processes. AI automates routine tasks, provides real-time data analysis, and offers predictive insights, enabling faster and more informed decision-making. This efficiency is essential for staying competitive in dynamic markets.
AI business models enterprise use cases
It is easy to consider why AI business models are helpful. However, examining use cases can illuminate how to implement them in your organization as we separate how their features benefit departments, beginning with finance.
Finance department
The finance department is a great place to start implementing AI due to the strengths and weaknesses of this technology.
AI is strong at processing large amounts of numerical data quickly, which forms the core of finance functions, and while AI is weak at being creative, this feature is not necessary in finance.
The ways AI business models can support the finance department are:
- Fraud detection: Utilizing AI to analyze transaction data and identify patterns indicative of fraudulent activities.
- Risk assessment: Leveraging AI models to evaluate credit risk and provide insights for informed lending decisions.
- Financial forecasting: Employing AI for predicting market trends and facilitating data-driven investment decisions.
- Regulatory compliance: Utilizing AI to ensure compliance with financial regulations through automated data checks and reporting.
HR department
AI can be instrumental in helping HR staff process many aspects of an employee’s journey quickly and efficiently, allowing the staff to focus on the soft skills of which AI is incapable.
Ways an AI business model can support HR include:
- Resume screening: Implementing AI to automate the initial screening of job applications, saving valuable time for HR teams.
- Employee onboarding: Utilizing AI chatbots to deliver information and guidance to new employees during onboarding.
- Employee engagement: Employing AI surveys and sentiment analysis to gauge employee satisfaction and extract valuable insights.
- Talent acquisition: Leveraging AI to match job candidates with relevant positions and identify top talent efficiently.
Operations department
An AI business model can help streamline operations to remove production gaps so products and services get to customers quickly and without delays.
Ways an AI business model can support operations include:
- Supply chain optimization: Using AI to manage inventory, facilitate demand forecasting, and plan logistics for optimal efficiency.
- Predictive maintenance: Implementing AI to predict equipment failures, reducing downtime and lowering maintenance costs.
- Quality control: Employing AI to identify defects and anomalies in manufacturing production processes, ensuring high-quality outputs.
- Customer service: Utilizing AI-powered chatbots and virtual assistants to handle customer inquiries and provide support.
And these applications of AI represent just the starting point. As technology advances, there will undoubtedly be new avenues for leveraging AI to enhance efficiency, accuracy, and quality across various industries and teams.
Add technology, but keep humans as the focus
In AI business models, the integration of cutting-edge technology harmonizes with a steadfast commitment to keeping humans at the forefront. These models prioritize enhancing customer experiences through personalized services, utilizing closed-loop processes for continuous improvement.
Asset sharing is optimized, fostering collaboration and sustainability. Usage-based pricing ensures flexibility and scalability, aligning with customer needs. Within collaborative ecosystems, innovation flourishes, expanding the reach of interconnected businesses.
An agile and adaptive organizational approach, empowered by AI, propels efficient decision-making and continuous learning, affirming that human-centric values remain paramount while technology evolves.