Artificial Intelligence (AI) encompasses all capabilities that enable machines to mimic human behaviors, particularly regarding cognitive functions. It’s about equipping machines with the essence of human-like decision-making and problem-solving skills.
While AI is a standalone concept, it’s intricately linked with disciplines such as Machine Learning (ML) and Data Science. In the contemporary landscape, AI has ascended to a pivotal role, poised to redefine efficiency and precision in various tasks.
Within AI, six critical branches underpin the creation of intelligent systems capable of tackling intricate challenges. Each branch brings distinct attributes to the table, coming together to fuel the sophisticated AI-driven solutions that are becoming increasingly prevalent.
The omnipresence of AI in our daily lives is subtle yet profound, and understanding these six branches is crucial to grasping the full impact AI will have on the future of technology.
By the end of this article, you will have an understanding of the following:
- What Artificial Intelligence (AI) is and the various stages it goes through
- The multiple types of AI, like the theory of mind AI
- The six main branches of artificial intelligence
What Is Artificial Intelligence (AI)?
Artificial Intelligence (AI) is a subfield of computer science that aims to create systems capable of performing tasks that would normally require human intelligence.
These tasks include pattern recognition, learning from experience, drawing conclusions, making predictions, or taking action to achieve specific goals. AI systems are powered by algorithms and fed by data, which they use to build a mathematical model of the world that allows them to act intelligently.
What Are The Stages Of AI?
Artificial Intelligence can progress through three stages of development rather than being categorized into types—which we’ll discuss in the next section.
Let’s delve deeper into each developmental phase of AI:
Artificial Narrow Intelligence (ANI)
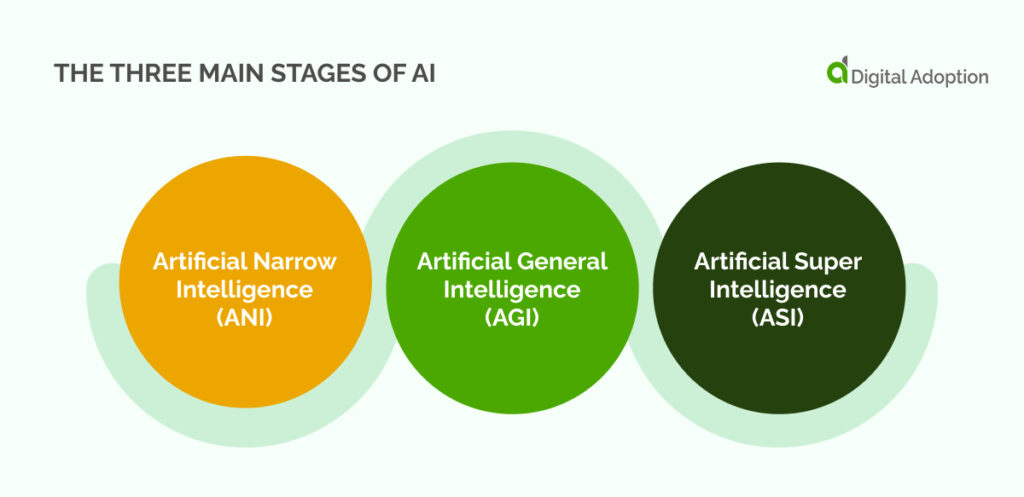
Commonly referred to as Weak AI, this initial stage of Artificial Intelligence encompasses systems designed to execute a narrowly confined array of tasks. At this juncture, machines lack genuine cognitive abilities and are programmed to follow a specific set of instructions.
Weak AI is evident in technologies like Siri, Alexa, autonomous vehicles, AlphaGo, and humanoid robots like Sophia. Presently, nearly all AI implementations are within this initial stage of development.
Artificial General Intelligence (AGI)
Often termed Strong AI, this evolutionary phase marks a period where machines can reason and make choices analogous to human intelligence.
While Strong AI has not yet been actualized, advancements suggest the possibility of developing machines with human-equivalent cognitive skills in the foreseeable future.
Artificial Super Intelligence (ASI)
This phase represents a point in AI development where computer intelligence will greatly exceed that of humans. ASI remains a speculative notion, predominantly portrayed in cinematic and literary works of science fiction where machines dominate humanity.
Given the rapid progression in this field, it’s conceivable to speculate that the emergence of ASI might not be a distant reality.
What Are The Types Of Artificial Intelligence?
The following types of AI all fall somewhere on the scale of different stages previously mentioned, particularly the former, where rudimentary developments characterize the current class of AI systems.
Along these different stages of development, emerging solutions can be categorized according to their operational capabilities.
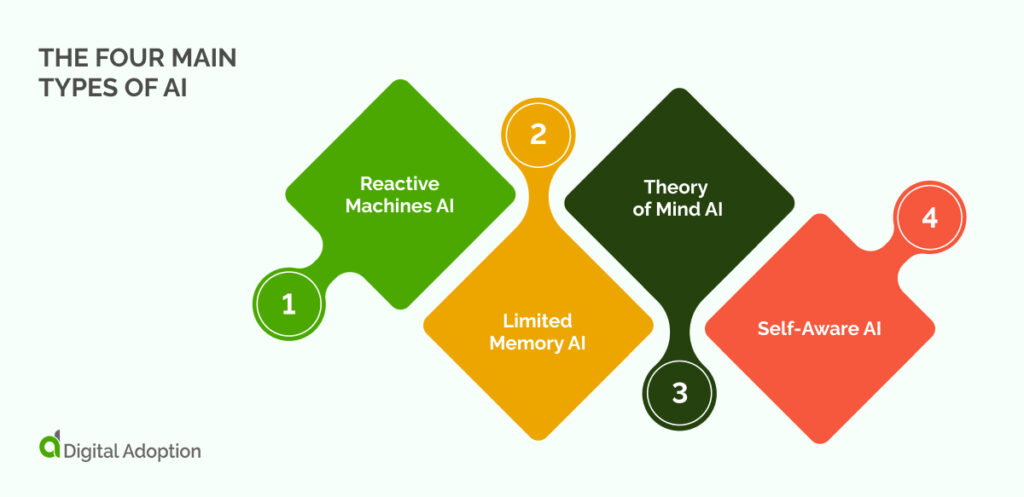
Here are the functional categories of AI systems:
Reactive Machines AI
This class of AI systems operates based on current data, addressing immediate tasks without the ability to use experience to influence future decisions. Reactive AI is programmed for a limited range of functions.
An illustrative example is IBM’s Deep Blue, the chess-playing computer that defeated the world champion Garry Kasparov.
Limited Memory AI
As the term suggests, this AI can learn from historical data to make better decisions. This kind of AI has transient memory that helps use previously gained information to inform future decisions.
Autonomous vehicles represent this category. They interpret sensory data to navigate safely, drawing from recent driving experiences to avoid obstacles or traffic.
Theory of Mind AI
This advanced AI category is still under research and aims to understand and interpret human emotions and mental states, potentially transforming interactions between technology and people.
Theory of Mind AI, while not yet realized, is being pursued to enable machines to understand and react to human emotions and thought processes.
Self-Aware AI
This type of AI, which involves machines gaining consciousness, is speculative and currently resides in science fiction. Self-aware AI would be capable of understanding and recognizing its existence and state of being.
6 Major Branches of Artificial Intelligence
Artificial Intelligence spans multiple specialties, including Machine Learning, Robotics, and many others.
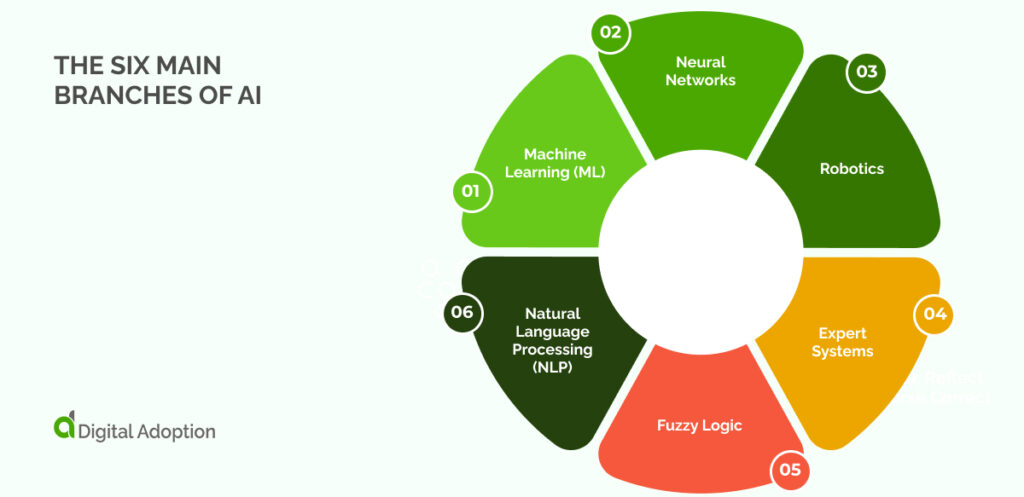
In this section, we will explore the six main branches of AI:
- Machine Learning (ML)
Machine Learning stands at the forefront of technological innovation, capturing attention with each novel application company’s launch. ML empowers systems to learn and improve from experience without explicit programming.
It’s become an integral part of our lives, often operating behind the scenes. ML is the scientific study that allows machines to process, interpret, and use data to solve real-world problems.
ML algorithms are crafted using advanced mathematical computations, encoded in machine-readable language, forming the backbone of a complete ML system. These algorithms enable deep learning systems to classify, decode, and predict outcomes from vast datasets.
Recently, ML has gifted us with autonomous vehicles, proficient image and speech recognition systems, predictive demand forecasting, enhanced internet searches, and various other sophisticated applications.
Its primary focus is developing applications that improve their decision-making and predictive capabilities over time through experience.
The choice of ML algorithm—supervised, unsupervised, or reinforcement learning—depends on the nature of the data at hand and the predictive tasks to be performed:
- Supervised Learning involves training algorithms with labeled data, specifying both the inputs and desired outputs, allowing them to find relationships between variables.
- Unsupervised Learning comprises algorithms that learn from unlabeled data, finding intrinsic patterns or groupings, like cluster analysis, which identifies hidden structures in data.
- Reinforcement Learning is used for tasks requiring a sequence of decisions, where algorithms learn to achieve a goal through rewards and penalties, sometimes autonomously deciding the next move.
- Neural Networks
Neural Networks merge cognitive function with computational models, drawing inspiration from the neurological structures of the human brain. These artificial neural networks imitate the brain’s interconnected neuron structure to process information and recognize patterns.
A neural network possesses layers of interconnected nodes or ‘artificial neurons.’ Each one is designed to perform specific mathematical operations. They use statistical methods, like regression analysis, to carry out tasks.
Neural networks are pivotal in fields ranging from finance in terms of fraud detection and risk management to sales forecasting and market research.
- Robotics
Robotics has rapidly evolved into a dynamic AI field, centering on creating robots. It’s a multidisciplinary arena involving mechanical engineering, electrical engineering, and computer science, among others.
Robots are created to perform tasks that may be repetitive or dangerous for humans. They’re prevalent in manufacturing assembly lines, space expeditions, and even social interaction settings, guided by machine learning algorithms.
- Expert Systems
One of the earliest successes in AI, expert systems, emerged in the 1970s and flourished in the 1980s. These systems emulate the decision-making ability of human experts by leveraging knowledge from a specific domain and applying inferential rules to user queries.
An expert system’s performance hinges on the richness of its knowledge base—the more comprehensive the knowledge, the more adept the system. These systems excel in providing spell-check suggestions or error identification, like those seen in Google’s search engine.
- Fuzzy Logic
Fuzzy logic provides individuals with a process for dealing with uncertain and imprecise information, assessing degrees of truth rather than the binary, true or false. It’s a way of processing data that reflects the complexities of real-world situations, allowing machines to emulate human reasoning more closely.
Fuzzy logic systems are beneficial where information is ambiguous or incomplete, providing a more nuanced approach to problem-solving.
- Natural Language Processing (NLP)
Natural Language Processing, a fascinating and challenging domain, is central to AI’s ability to interact fluently with human languages. NLP involves computational techniques for the processing and understanding of human speech, allowing computers to interpret text and spoken words in a valuable way.
NLP facilitates various applications, including text summarization, machine translation, and sentiment analysis. It is also the technology behind personal digital assistants and has enabled advancements in customer service through chatbots and improved user experience by interpreting customer feedback.
Key Takeaways Related To Branches Of Artificial Intelligence
As we navigate through the burgeoning AI landscape, the advancements in AI are embedding themselves more deeply into the fabric of our everyday lives. For example, bleeding-edge NLP capabilities have led to the emergence of ChatGPT—a radical AI-powered chatbot reimagining the means of workplace productivity.
In time, the notion of manually programming computers could become obsolete as they gain the ability to engage in dialogue with us and learn autonomously from ongoing streams of data. This paradigm shift can redefine our interactions with digital systems, enabling computers to shoulder increasingly intricate tasks easily.
The spectrum of AI encompasses diverse approaches, each tailored to tackle complex problems and distill meaningful insights from vast datasets.
Each AI discipline possesses unique advantages and constraints. The optimal approach for a specific task necessitates meticulous analysis involving an in-depth assessment of the task’s particular prerequisites and limitations to fully harness AI’s potential for a specific use case.
For example, fuzzy logic is employed in various domains, including modifying vehicle braking systems based on parameters like acceleration, velocity, and wheel rotation rate. Conversely, Natural Language Processing (NLP) equips machines with the capacity to understand human language, both spoken and written.
This proficiency is exploited in numerous applications, ranging from driving conversational chatbots and spam filtering to scrutinizing textual sentiment and more.